How do VR headsets work?? Lets talk Production, Encoding, Transmission and Decoding of 3D images
Production
3D movies/images trick your brain by bringing images projected onto a flat cinema screen to life in full three dimensional glory.
If you look at an object near you and close your left and right eyes in turn, you'll see that each has a slightly different view of the world. Your left eye sees a bit more of the left side of the object, and your right eye sees a bit more of its right side. Your brain fuses the two images together allowing you to see in three dimensions. This is known as stereoscopic vision.
To create a similar effect, 3D films are captured using two lenses placed side by side, just like your eyes (or by producing computer generated images to replicate the same effect).
In old fashioned 3D films, footage for the left eye would be filmed using a red lens filter, producing a red image, and footage for the right eye would be shot using a blue filter, resulting in a blue image. Two projectors then superimposed the images on the cinema screen.
3D glasses with blue and red filters ensured viewers' left and right eyes saw the correct image: the red filter would only let red light through to your left eye, and the blue filter would only let blue light through to your right eye. Your brain would then combine these two slightly different images to create the illusion of 3D. Unfortunately, this meant that old fashioned 3D films couldn't make full use of colour.
To get around this problem, modern 3D films use polarised light instead of red and blue light.
What is polarised light?
A polarised light wave vibrates on only one plane. The light produced by the sun is unpolarised, meaning it is made up of light waves vibrating on many different planes. It can however be transformed into polarised light using a polarising filter.
A polarising filter has tiny parallel lines etched into it, a bit like the slats on a set of venetian blinds. This means it will only let light vibrating on a particular plane through.
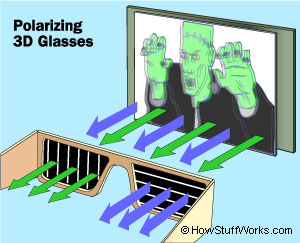
As with old fashioned 3D, the film is recorded using two camera lenses sat side by side. But in the cinema, the two reels of film are projected through different polarised filters. So images destined for viewers' left eyes are polarised on a horizontal plane, whereas images destined for their right eyes are polarised on a vertical plane.
Cinema goers' glasses use the same polarising filters to separate out the two images again, giving each eye sees a slightly different perspective and fooling the brain into 'seeing' Avatar's planet Pandora as though they were actually there.
Why aren't 3-D glasses red and blue anymore?
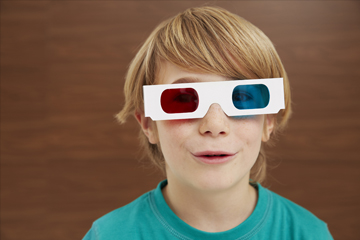
3-D cinema. The first 3-D movie, "The Power of Love," screened at the Los Angeles Ambassador Hotel in 1922. To create the 3-D effect, two films were projected simultaneously while the audience watched through stereoscopes, which looked a lot like the toy View-Master you might have had as a child.
3-D movies didn't reach mass appeal until the 1950s, mostly with the cult and horror genres. This is when the white cardboard glasses with red and blue lenses came into vogue -- the technical name is anaglyph. Despite anaglyph glasses' universal association with 3-D, they're rarely used anymore.
The traditional 3-D anaglyph glasses use one red lens and one blue (cyan) lens. Many other color combinations work, too, like red and green, but red and blue were used most frequently. The blue lens filters out all the red light, and the red lens filters out all the blue light, so each eye sees a slightly different image.
When the 3-D movie is projected on the screen, two images are displayed: one in red, one in blue. Since each lens of the glasses has a filter, only one image can reach each eye. The brain merges both images together, which results in the illusion of an image popping or jumping off the screen.
However, using the red and blue color combination to get a sensation of depth also causes a degradation of color in the movie. The image quality is a bit fuzzy, and the color of the 3-D film always looks a little off. The industry responded with polarized 3-D lenses to create a better experience for moviegoers.
Polarized 3-D glasses don't rely on separately colored lenses to get the 3-D effect. They work a lot like anaglyph glasses, though, by tricking the brain into merging two images into one. Polarized lenses have a yellow-brown tint instead of red and blue. When light is polarized, all the waves oscillate in one direction. The camera projects one image that polarizes vertically, and another polarizes horizontally. Each lens only lets through one kind of image, and your brain merges each image into one. The end result? Crisp 3-D images with very little degradation in color or quality!
Compression
Why even compress anything?
A simple uncompressed video file will contain an array of 2D buffers containing pixel data for each frame. So it's a 3D (2 spatial dimensions and 1 temporal) array of bytes. Each pixel takes 3 bytes to store - one byte each for the three primary colors (red, green and blue).
1080p @ 60 Hz = 1920x1080x60x3 => ~370 MB/sec of raw data.
This is next to impossible to deal with. A 50GB Blu-ray disk will only hold ~2 mins. You can't move it anywhere fast.
So yeah. We need compression.
Why H.264 compression?
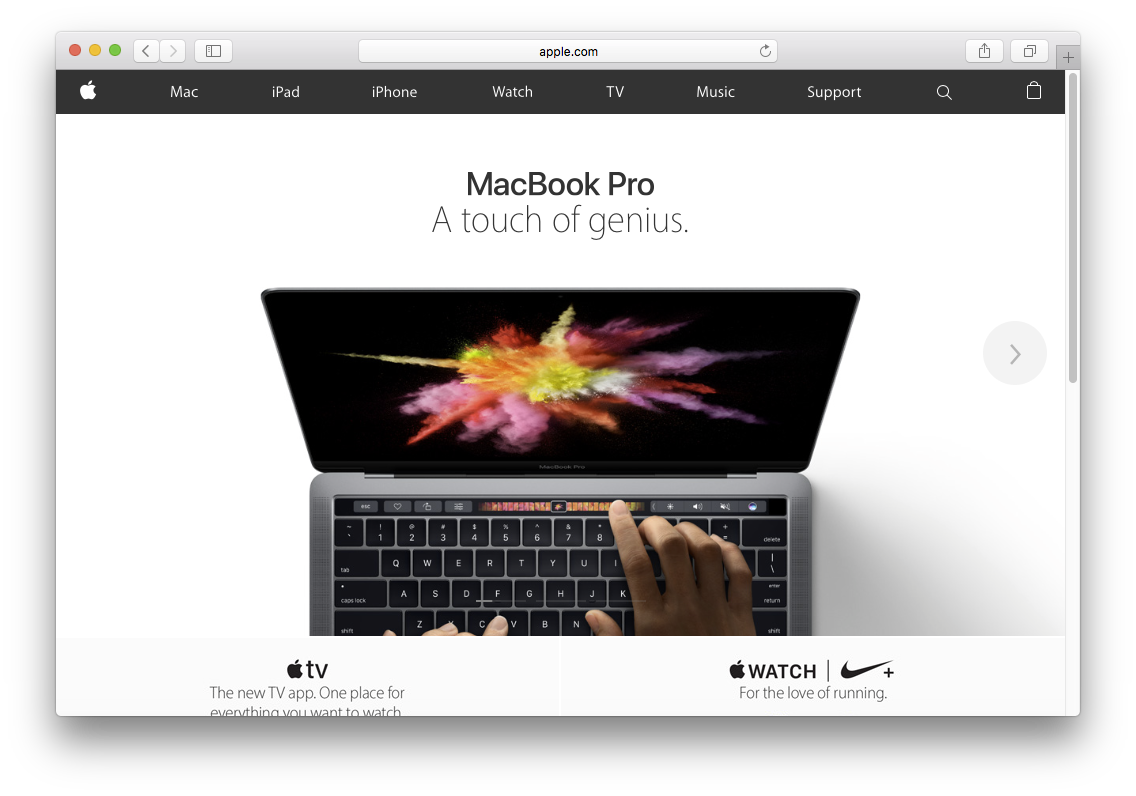
It has been reproduced as
Eh. What? Those file sizes look switched.
No, they're right. The H.264 video, 300 frames long is 175KB. A single frame of that video in PNG is 1015KB.
It looks like we're storing 300 times the amount of data in the video. But the file size is a fifth. So H.264 would seem to be 1500x as efficient as PNG.
How is this even possible? All right, what's the trick?
There are very many tricks! H.264 uses all the tricks you can think of (and tons you can't think of). Let's go through the important ones.
Shedding weight
Imagine you're building a car for street racing. You need to go faster. What is the first thing you do? You shed some weight. Your car weighs 3000 lbs. You throw away stuff you don't need. Those back seats? pfft. Chuck those. That subwoofer? Gone. No music for you. Air Conditioning? Yeah, ditch it. Transmission? Ye..no. Wait! We're gonna need that.
You remove everything except the things that matter.
This concept of throwing away bits you don't need to save space is called lossy compression. H.264 is a lossy codec - it throws away less important bits and only keeps the important bits.
PNG is a lossless codec. It means that nothing is thrown away. Bit for bit, the original source image can be recovered from a PNG encoded image.
There are few obvious ways to trim out images. Maybe the top right quadrant is useless all the time. So maybe we can zero out those pixels and discard that quadrant. We would use only 3/4th of the space we need. ~2200 lbs now. Or maybe we can crop out a thick border around the edges of the frame, the important stuff is in the middle anyway. Yes, you could do these. But H.264 doesn't do this.
What does H.264 actually do?
H.264, like other lossy image algorithms, discards detail information. Here is a close-up of the original compared with the image post-discard.
Important bits? How does the algorithm know what bits in my frame are important?
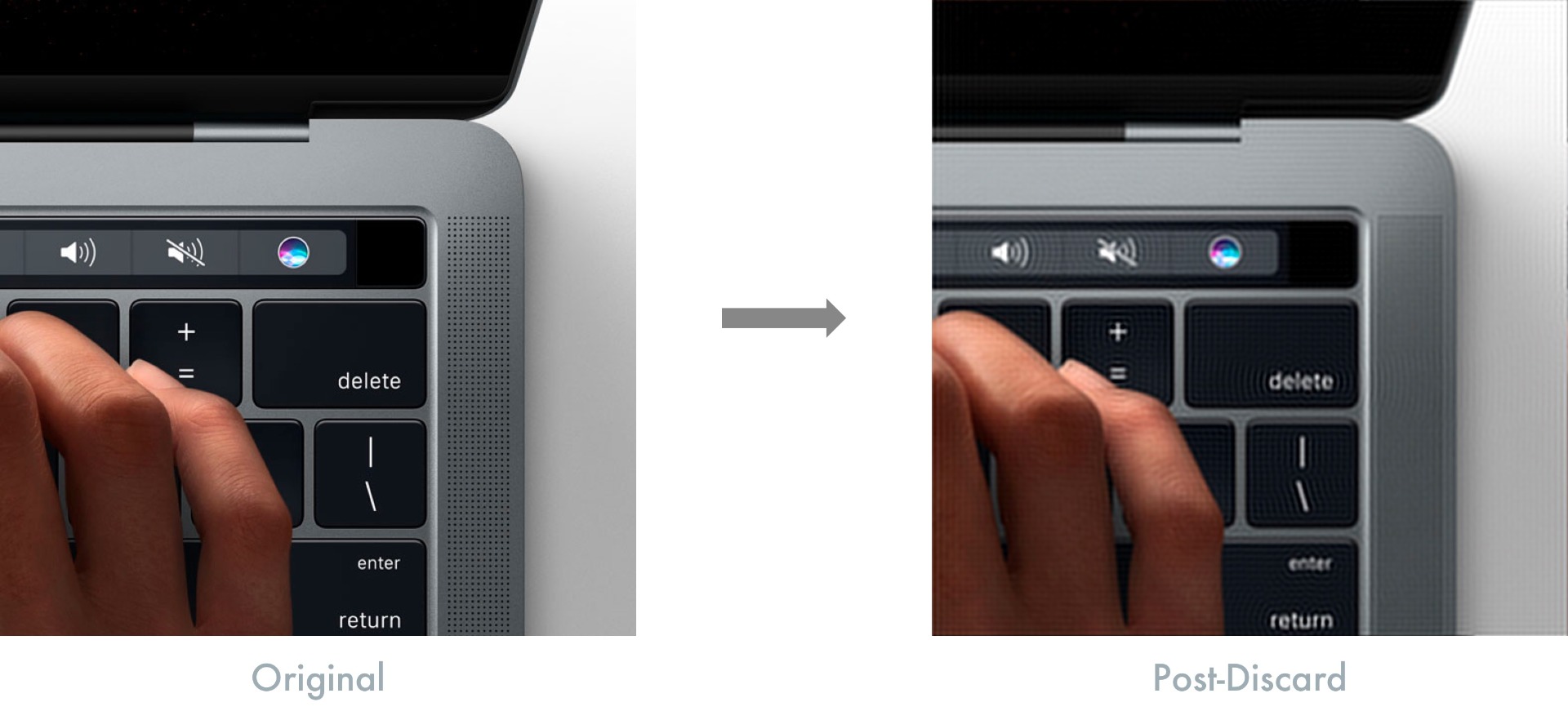
See how the compressed one does not show the holes in the speaker grills in the MacBook Pro? If you don't zoom in, you would even notice the difference. The image on the right weighs in at 7% the size of the original - and we haven't even compressed the image in the traditional sense. Imagine your car weighed just 200 lbs!
For this we need a quick math lesson.
Information Entropy
If you took an information theory class, you might remember information entropy. Information entropy is the number of bits required to represent some information. Note that it is not simply the size of some dataset. It is minimum number of bits that must be used to represent all the information contained in a dataset.
For example, if your dataset is the result of a single coin toss, you need 1 bit of entropy. If you have record two coin tosses, you'll need 2 bits. Makes sense?
Suppose you have some strange coin - you've tossed it 10 times, and every time it lands on heads. How would you describe this information to someone? You wouldn't say HHHHHHHHH. You would just say "10 tosses, all heads" - bam! You've just compressed some data! Easy. I saved you hours of lectures. This is obviously an oversimplification, but you've transformed some data into another shorter representation of the same information. You've reduced data redundancy. The information entropy in this dataset has not changed - you've just converted between representations. This type of encoder is called an entropy encoder - it's a general-purpose lossless encoder that works for any type of data.
Frequency Domain
Now that you understand information entropy, let's move on to transformations of data. You can represent data in some fundamental units. If you use binary, you have 0 and 1. If you use hex, you have 16 characters. You can easily transform between the two systems. They are essentially equivalent. So far so good? Ok!
Now, some imagination! Imagine you can transform any dataset that varies over space(or time) - something like the brightness value of an image, into a different coordinate space. So instead of x-y coordinates, let's say we have frequency coordinates. freqX and freqY are the axes now. This is called a frequency domain representation. There is another mathematical theorem[1] that states that you can do this for any data and you can achieve a perfect lossless transformation as long as freqX and freqY are high enough.
Okay, but what the freq are freqX and freqY?
freqX and freqY are some other set of basis units. Just like when we switch from binary to hex, we have a different fundamental unit, we're switching from the familiar X-Y to freqX and freqY. Hex 'A' looks different from binary '1010'. Both mean the same thing, but look different.
Chroma Subsampling.
The human/eye brain system is not very good at resolving finer details in color. It can detect minor variations in brightness very easily but not color. So there must be some way to discard color information to shed even more weight.
In a TV signal, R+G+B color data gets transformed to Y+Cb+Cr. The Y is the luminance (essentially black and white brightness) and the Cb and Cr are the chrominance (color) components. RGB and YCbCr are equivalent in terms of information entropy.
Why unnecessarily complicate? RGB not good enough for you?
Back before we had color TV, we only had the Y signal. And when color TVs just started coming along, engineers had to figure out a way to transmit RGB color along with Y. Instead of using two separate data streams, they wisely decided to encode the color information into Cb and Cr and transmit that along with the Y information. That way, Black and White TVs would only look at the Y component. Color TVs will, in addition, look at the chrominance components and convert to RGB internally.
But check out the trick: the Y component gets encoded at full resolution. The C components only at a quarter resolution. Since the eye/brain is terrible at detecting color variations, you can get away with this. By doing this, you reduce total bandwidth by one half, with very little visual difference. Half! Your car now weighs 30 lbs!
This process of discarding some of the color information is called Chroma Subsampling[2]. While not specific to H.264 and has been around for decades itself, it is used almost universally.
Those are the big weight shedders for lossy compression. Our frames are now tiny - since we discarded most of the detail information and half of the color information.
Wait. That's it? Can we do something more?
Yes. Weight shedding is only the first step. So far we're only looking at the spatial domains within a single frame. Now it's time to explore temporal compression - where we look at a group of frames across time.
Motion compensation
H.264 is a motion compensation compression standard.
Motion compensation? What now?
Imagine you're watching a tennis match. The camera is fixed at a certain angle. The only thing moving is the ball back and forth. How would you encode this information? You do what you always do, right? You have a 3D array of pixels, two dimensions in space and one in time. Right?
Nah. Why would you? Most of the image is the same anyway. The court, the net, the crowds, all are static. The only real action is the ball moving. What if you could just have one static image of everything in the background, and then one moving image of just the ball? Wouldn't that save a lot of space? You see where I am going with this? Get it? See where I am going? Motion estimation?
Lame jokes aside, this is exactly what H.264 does. H.264 splits up the image into macro-blocks - typically 16x16 pixel blocks that it will use for motion estimation. It encodes one static image - typically called an I-frame(Intra frame). This is a full frame - containing all the bits it required to construct that frame. And then subsequent frames are either P-frames(predicted) or B-frames(bi-directionally predicted). P-frames are frames that will encode a motion vector for each of the macro-blocks from the previous frame. So a P-frame has to be constructed by the decoder based on previous frames. It starts with the last I-frame in the video stream and then walks through every subsequent frame - adding up the motion vector deltas as it goes along until it arrives at the current frame.
B-frames are even more interesting, where the prediction happens bi-directionally, both from past frames and from future frames. So you can imagine now why that Apple home page video is so well compressed. Because it's really just three I-frames in which the macro blocks are being panned around.
Let's say you've been playing a video on YouTube. You missed the last few seconds of dialog, so you scrub back a few seconds. Have you noticed that it doesn't instantly start playing from that timecode you just selected. It pauses for a few moments and then plays. It's already buffered those frames from the network, since you just played it, so why that pause?
Yeah that annoys me. Why does it do that?
Because you've asked the decoder to jump to some arbitrary frame, the decoder has to redo all the calculations - starting from the nearest I-frames and adding up the motion vector deltas to the frame you're on - and this is computationally expensive, and hence the brief pause. Hopefully you'll be less annoyed now, knowing it's actually doing hard work and not just sitting around just to annoy you.
Since you're only encoding motion vectors deltas, this technique is extremely space-efficient for any video with motion, at the cost of some computation.
Now we've covered both spatial and temporal compression! So far we have a lot of space saved in Quantization. Chroma subsampling further halved the space required. On top of that, we have motion compensation that stores only 3 actual frames for the ~300 that we had in that video.
Looks pretty good to me. Now what?
Now we wrap up and seal the deal. We use a traditional lossless entropy encoder. Because why not? Let's just slap that on there for good measure.
Entropy Coder
The I-frames, after the lossy steps, contain redundant information. The motion vectors for each of the macro blocks in the P and B-frames - there are entire groups of them with the same values - since several macro blocks move by the same amount when the image pans in our test video.
An entropy encoder will take care of this redundancy. And since it is a general purpose lossless encoder, we don't have to worry about what tradeoffs it's making. We can recover all the data that goes in.
And, we're done! At the core of it, this is how video compression codecs like H.264 work. These are its tricks.
Ok great! But I am curious to know how much our car weighs now.
The original video was captured at an odd resolution of 1232x1154. If we apply the math here, we get:
5 secs @ 60 fps = 1232x1154x60x3x5 => 1.2 GB
Compressed video => 175 KB
If we apply the same ratio to our 3000 lb car, we get 0.4 lbs as the final weight. 6.5 ounces![3]
Yeah. It's magic!
References
- Nyquist-Shannon Sampling Theorem
- Chroma Subsampling
- https://sidbala.com/h-264-is-magic/